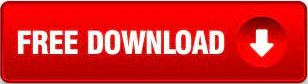
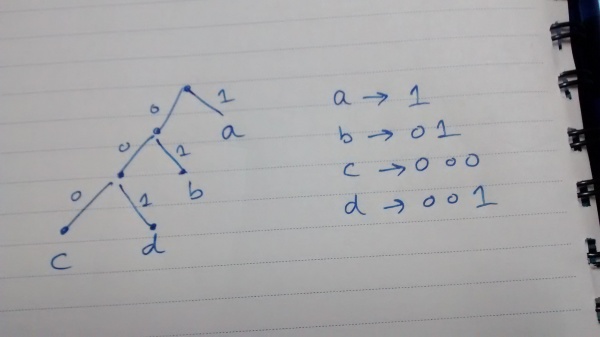
#GIVEN A WORD BUILD HUFFMAN CODE TREE FULL#
In this case, we want to keep the full words in tact. In typical applications of the Huffman coding scheme, we’d be encoding characters. Note that we’re encoding full words here. So now we have a nice Huffman tree that provides binary codes for each full word in the vocabulary. Here’s what the tree looks like in tree form: Or you can just skip the excitement and the fun and click on “end” to skip to the final state of memory after this part of the process. Try to follow the code above and step through this to understand how we’re building the tree.
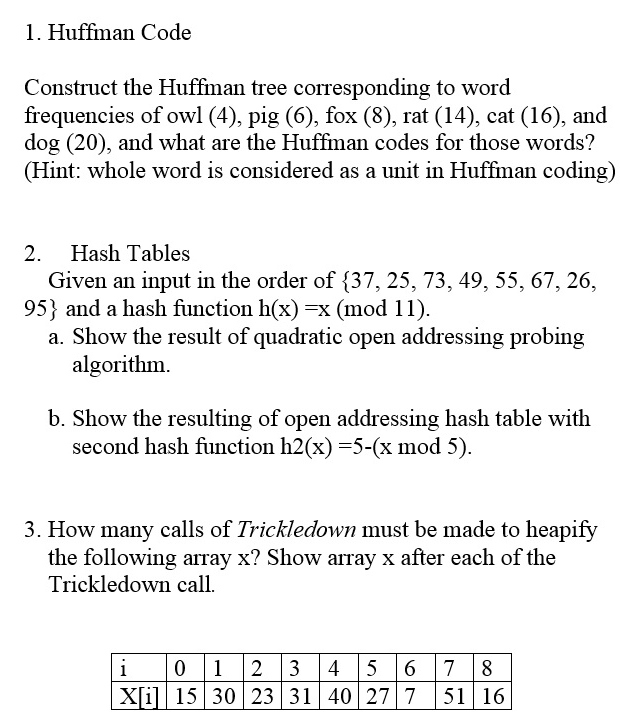
You can either click “play” and watch the magic happen, or you can click “next” for each step through of each change in memory in the algorithm. The “grayer” columns (indices 9 to 18) are used for non-leaf nodes in the tree. Each white column in this table represents one of our 8 words. Step through the below animation for a walkthrough of how this works in our “wood chuck” example. The magic is in the source code: void CreateBinaryTree () part 1: tree construction word2vec’s CreateBinaryTree() I wrote this post to explain what I found. īefore reading about word2vec, I was familiar with Huffman coding as a means of lossless data compression, but I was confused about how exactly the tree is constructed, and then how it is used in word2vec’s “hierarchical softmax” method. For the “hierarchical softmax” method, a Huffman binary tree is used. One is the so called “hierarchical softmax” and the other is a process called “Noise Contrastive Estimation”. There are two major approaches to training the word2vec model. We don't have to keep sorting the queue after every insert, rather have a look at a proper priority queue.How does the huffman tree work in word2vec? We can do a full traversal once and keep track of each character and its Huffman code in a map.Īlso building up the queue can be improved. For example, when encoding the text and looking up the Huffman code in the tree, we don't have to walk the tree for every character. There's several improvements we can make to this implementation. reduce ( >, fn character, binary -> code = find ( tree, character ) > end ) end end Replace the contents of the file with a single function declaration encode/1 like so:ĭefp build ([, character, path ) do find ( left, character, > ) || find ( right, character, > ) end defp convert ( text, tree ) do text |> String. cd huffman into the project root and open up the lib/huffman.ex file. Ok! Let's begin by generating a new Mix project using mix new huffman. Let's get to work: Setting up the project Once you hit a character, write it down and start over with the remainder of the bits.įor an excellent explanation on the Huffman Coding algorithm, give this explainer video by Tom Scott a watch! Repeat the process for each character in the text, and voila!ĭecoding the text is as simple as starting at the root of the tree and traverse down the left-child for every 0 you encounter and pick the right-child for every 1. Every time we pick the left-child, we write down a 0 and for every right-child a 1. The Huffman code can be derived by walking the tree until we find the character. Placing characters with a higher frequency closer to the root of the tree than characters with a lower one. The algorithm in its core works by building a binary tree based on the frequency of the individual characters. In this example we assume the data we are compressing is a piece of text, as text lends itself very well for compression due to repetition of characters. In this post we walk through implementing a Huffman coder and decoder from scratch using Elixir ⚗️ It utilizes a binary tree as its base and it's quite an easy to grasp algorithm. Huffman coding is a pretty straight-forward lossless compression algorithm first described in 1992 by David Huffman.
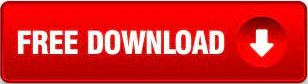